On the 21st of December 2024, students pursuing the Master’s degree in Electronics Science and Technology, an F2F-Hybrid joint Program between the Department of Electrical Engineering, Computer Engineering and Informatics at Cyprus University of Technology and the Hangzhou Dianzi University, in China, presented a short overview of the work they have completed, so far. Two students are supervised by one of the leaders of the ehealthlab@CUT, the Adjunct Prof. Christos Loizou.
Below, we share information on their on-going MSc Degree projects.
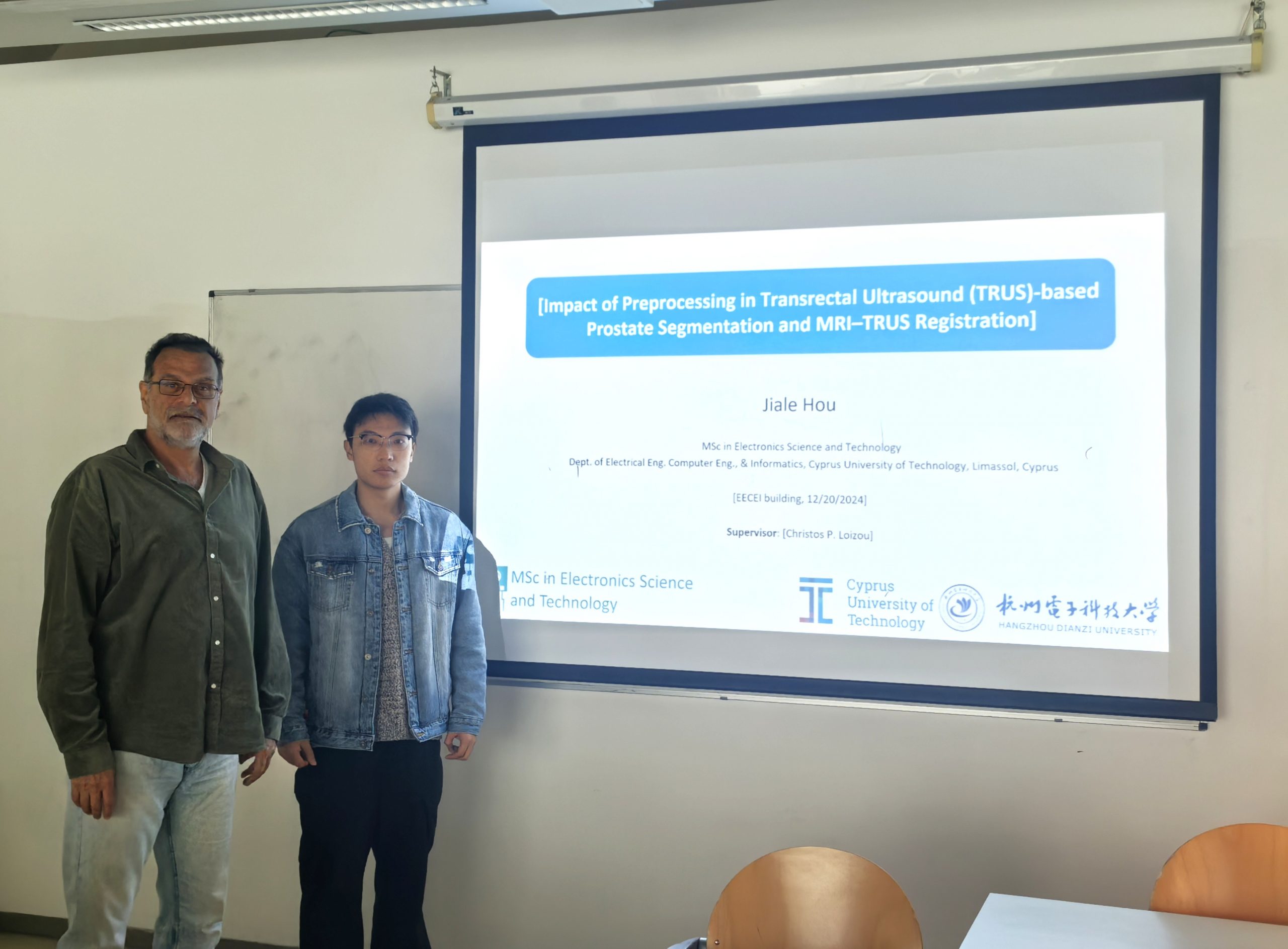
Jiale W. Hou
Impact of preprocessing in TRUS‐based prostate segmentation and MRI–TRUS registration
Abstract
This study aims to enhance prostate segmentation in Transrectal Ultrasound (TRUS) axial images, by leveraging image preprocessing strategies, prior to deep learning model utilization, such as image intensity normalization, denoising filtering, and the combination of both. TRUS and Magnetic Resonance Imaging (MRI) sets of images (9 patients), were provided (with manual annotations of the regions of interest in TRUS), by doctors at German Medical Institute, in Cuprus. The TRUS images with prostate efficiently segmented will be registered and fused with the MRI images. Next, with a similar approach, we will test preprocessing methods to improve prostate segmentation in MRI. The results are expected to show a positive impact of preprocessing in prostate segmentation in both TRUS and MRI images, during brachytherapy.
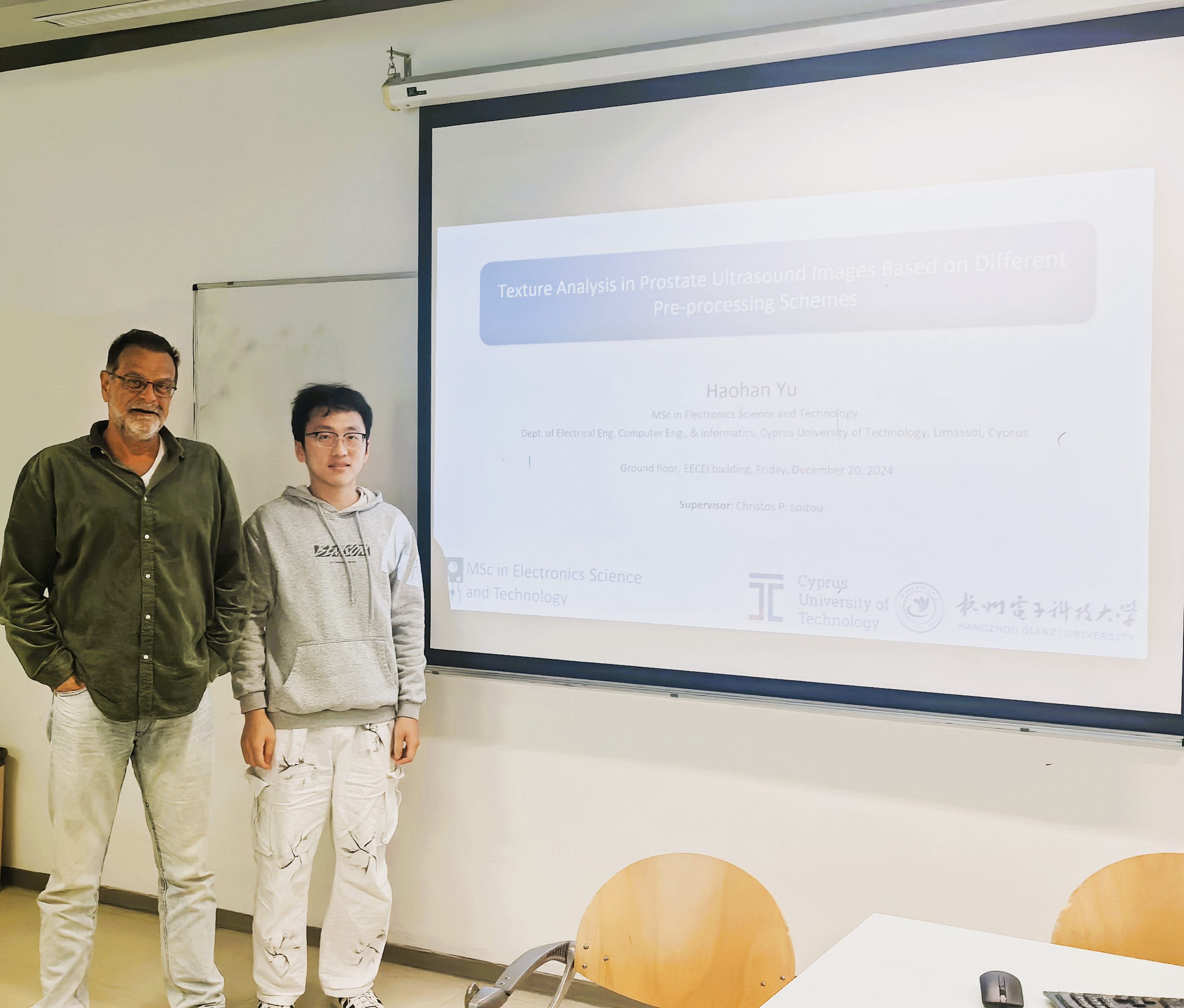
Yu Haohan
Texture Analysis in Prostate Ultrasound Images Based on Different Pre-processing Schemes
Abstract
Prostate cancer (PCa) is a significant health concern for men worldwide, ne-cessitating reliable diagnostic tools to improve early detection and prognosis. This study focuses on enhancing the diagnostic accuracy of transrectal ultra-sound (TRUS) images for PCa by evaluating the impact of various image preprocessing techniques, including normalization, median filtering, bilateral filtering, Wiener filtering, and non-local means filtering. A dataset of ultra-sound images from ten patients was processed to mitigate noise and optimize texture feature extraction. Texture analysis methods, such as Gray-Level Co-occurrence Matrix (GLCM) and Wavelet-GLSZM, were employed to identify robust features for classification. The results revealed that non-local means filtering (NLMF) outperformed other techniques in noise reduction and image detail preservation, achieving superior metrics for PSNR, SSIM, and CNR. Certain texture features, such as WHLHGLSH, exhibited high stability across preprocessing methods, making them suitable for distinguishing between cancerous and non-cancerous tis-sues. In contrast, features like GLCI showed greater sensitivity to noise, lim-iting their reliability.
This study provides a systematic framework for improving TRUS image analysis and highlights the critical role of preprocessing in enhancing diag-nostic accuracy. Future directions include integrating MRI for multi-modal analysis and expanding the study to large-scale, multicenter datasets for diagnostic validation.